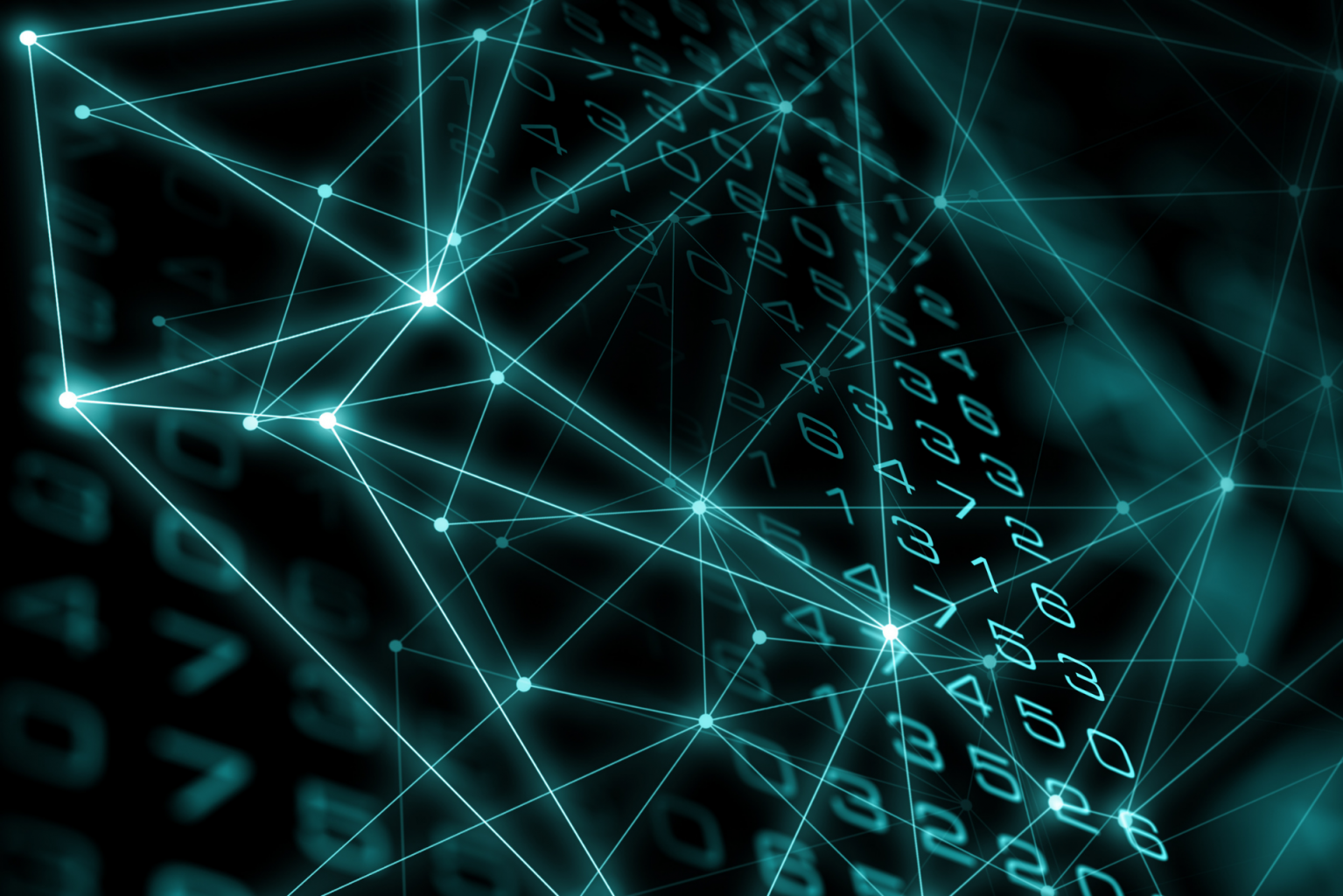
At a glance
Title | Distributed Machine Learning Solutions for Coordinating Distributed Energy Resources at the Edge of the Power Grid |
Reference | UTAP-EXPL/CA/0065/2021 |
Scientific Area | Advanced Computing |
Funding (PT) | 49 256,6 EUR |
Funding (US) | 50 000 USD |
Leading Institutions | Institute for Systems and Robotics, PT |
Duration | |
Start date | March 1, 2022 |
End date | |
Keywords | Collaborative Learning, Distributed Computation, Transactive Energy, Community Microgrids |
What is ML@GridEdge about?
The aging electric grid infrastructure has to deal with (i) increased penetration of intermittent energy assets (e.g., solar PV), (ii) rising expectations of end-use customers (e.g., seamless EV charging experience), and (iii) adverse impact of climate change.
Smart buildings and connected communities are the cornerstones of future sustainable power grids. Tomorrow’s energy communities and the resilient operation of the energy infrastructure hinges on accurate prediction of buildings’ temporal energy presumption (production and consumption). Traditional prediction models primarily leverage historical information (such as energy demand) at the grid or building level. This is mainly due to data sharing hesitance which originates from privacy concerns of building managers and occupants.
This project intends to develop a novel distributed machine learning model for predicting the temporal energy needs of future connected communities. The proposed multi-agent approach leverages centralized oversight of a central aggregator to inform distributed collaboration among each building, taking advantage of additional private information that is not shared with the aggregator. The proposed solution lends itself well to a multi-agent structure of connected energy communities where agents are willing to collaborate to improve their prediction accuracy.
What critical challenges is ML@GridEdge addressing?
Increasing renewable generation adoption by buildings and communities increases variability and challenges traditional energy management paradigms. Rapid decarbonization relies on integrating renewable sources with the energy supply chain. Accurate prediction of end-use energy needs and self-production patterns is the key to the desired integration. However, traditional approaches for predicting electricity consumption and production are inadequate to achieve such an objective.
This proposal intends to address this gap by creating a collaborative prediction solution that improves prediction accuracy at the system level while preserving end-user customers’ privacy.
What does ML@GridEdge propose to make the prediction of end-use energy more accurate?
This project intends to use a novel federated learning model for predicting building temporal presumption in transactive energy communities. The centralized coordinator (aggregator) has access to the historical net-metering profiles of the agents (buildings) and uses this information for feature extraction. The selected buildings locally compute an update to the model by executing the training program using their private data. Then, the aggregator collects the aggregated data and locally updates the shared model. Therefore, clients maintain their private data as confidential and simultaneously contribute to improving the prediction in their building and the community.
How is ML@GridEdge likely to contribute to the decarbonization of society?
The results of the developed models will ensure reliable and detailed previsions of the temporal presumption in buildings and communities. Such information will be crucial for optimizing energy resources in communities and smart cities. Put differently, data sharing empowers effective management of energy resources (such as battery energy storage and electric vehicles) and ensures economic and technical benefits for buildings, communities, and utilities.
This will provide an effective transition to future decarbonized energy systems with large-scale integration of renewable energy generation while ensuring a high-reliability level in the electric grid infrastructure.
Expected Key Outcomes
- Development of a collaborative energy prediction solution to address a significant obstacle to the increased adoption of renewable energy sources;
- Development of models and related software applications;
- Dissemination of results through conference, journal papers, and public reports;
- Dissemination of knowledge resulting from the project through courses offered at UT Austin and the University of Coimbra;
- Training of several MSc and Ph.D. students.
Papers and Communications
- Almeida, L., Soares, A., & Moura, P. (2023). A Systematic Review of Optimization Approaches for the Integration of Electric Vehicles in Public Buildings. In Energies (Vol. 16, Issue 13, p. 5030). MDPI AG. https://doi.org/10.3390/en16135030
- Correia, A. F. M., Lopes, J. M. B., Coimbra, A. P., Moura, P., & Almeida, A. T. de. (2023). Architecture and Control Simulation for Improved System Resiliency of a Building Microgrid. In 2023 IEEE/IAS 59th Industrial and Commercial Power Systems Technical Conference (I&CPS). 2023 IEEE/IAS 59th Industrial and Commercial Power Systems Technical Conference (I&CPS). IEEE. https://doi.org/10.1109/icps57144.2023.10142074
- Nelson, K., Moura, P., & Mohammadi, J. (2023). EVs and ERCOT: Foundations for Modeling Future Adoption Scenarios and Grid Implications. In 2023 11th Workshop on Modelling and Simulation of Cyber-Physical Energy Systems (MSCPES). 2023 11th Workshop on Modeling and Simulation of Cyber-Physical Energy Systems (MSCPES). IEEE. https://doi.org/10.1109/mscpes58582.2023.10123430
- Moura, P., Mohan, A., Lau, S., & Mohammadi, J. (2023). Maximizing Decarbonization Benefits of Transportation Electrification in the U.S. In Electricity (Vol. 4, Issue 1, pp. 46–61). MDPI AG. https://doi.org/10.3390/electricity4010004
- Correia, A. F. M., Moura, P., & de Almeida, A. T. (2022). Technical and Economic Assessment of Battery Storage and Vehicle-to-Grid Systems in Building Microgrids. In Energies (Vol. 15, Issue 23, p. 8905). MDPI AG. https://doi.org/10.3390/en15238905
- Mendes, N., Moura, P., Mendes, J., Salles, R., & Mohammadi, J. (2022). Federated Learning Enabled Prediction of Energy Consumption in Transactive Energy Communities. In 2022 IEEE PES Innovative Smart Grid Technologies Conference Europe (ISGT-Europe). 2022 IEEE PES Innovative Smart Grid Technologies Conference Europe (ISGT-Europe). IEEE. https://doi.org/10.1109/isgt-europe54678.2022.9960350
- Soares, A., Goncalves, G., & Moura, P. (2022). Management of Energy Storage in Transactive Energy Communities. In 2022 International Conference on Smart Energy Systems and Technologies (SEST). 2022 International Conference on Smart Energy Systems and Technologies (SEST). IEEE. https://doi.org/10.1109/sest53650.2022.9898494
Project Team
Pedro Moura
ML@GridEdge
Javad Mohammadi
ML@GridEdge